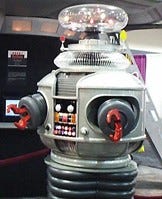
[Part of TSI’s on-going blog co-authoring effort, this blog was developed with Paul Grote, VP Business Partner Management at HUB International]Danger Will Robinson!
B9, the Robinson Robot, “Lost in Space”
Artificial Intelligence (AI) covers a spectrum of technologies. Some of those have been on an upward trend in the last few months. The tech is making a bit of a post-pandemic comeback thanks to the magic of ChatGPT and the financial performance of Nvidia. We remember the excitement back in 2018, when robotic process automation (RPA) was in the news and the top “push” service offering from all the key consulting firms. Five years later, Artificial Intelligence is back with fervor. Is AI really all that great? Why the ups and downs of interest in the market?
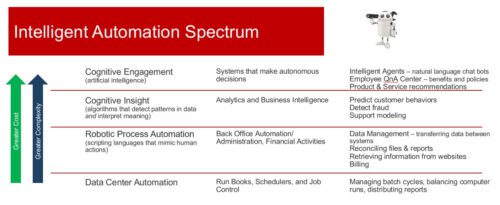
The airplane, the Smartphone, Artificial Intelligence
The common denominator for the three items above is that they are all responsible for sea change shifts, not only in technology, but in our culture and the way we live day today. The authors have no doubt that AI is here to stay and will have a significant impact. It will change the way we live and work. However, we do not believe that the change is quite here yet. Globally, we are just testing it out (think more the Wright Brothers than the Right Stuff).
Companies that can afford the investments will continue to experiment. 70% of respondents to an S&P Global Trends in AI survey said they had at least one AI project in production. One of the challenges in trying to leverage these powerful tools is that enterprise data is not as well organized as it needs to be, as noted by Axios in their summary of the S&P report. If one does not have data, there is nothing for AI to leverage.
Some companies do have good data. McKinsey and Company recently debuted an internal chat application for employees. That is a milestone for AI. However, after tours in professional services firms, the authors know that management consulting firms are comparatively ahead of the curve in terms of well-organized data. They tend to have extensive, well-indexed, and highly managed document repositories. These “knowledge bases” are often the key for global services firms’ ability to share and reuse materials to their advantage. And now the information they contain is critical in the AI race for effectiveness. Unlike McKinzie, most companies do not have well curated databases of information managed by a specialized knowledge management team.
Acquiring good data is not easy. For example, one HR organization we know was going to use a web chat-bot to improve employee experience and improve responsiveness of the HR team. But the team needed key data, decision rules, codified processes and advice, and workflow to feed the chat-bot. Since the team had little in the way of formal documentation, the AI project was scrapped. Leadership estimated it would have taken over a year to build the knowledge base for the AI to inquire against.
Many organizations struggle with data management for more traditional applications, such as operational reporting and business intelligence reports. In a recent post about current state analysis, we discussed that defining data exchange requirements between just two systems is a detail-oriented and time-consuming exercise. The data management challenges for enterprise AI go well beyond this simple example. It is unrealistic to expect most organizations to quickly make the jump to next-level data management capabilities that are demanded by truly impactful AI applications.
Discovering Fire
As if the data management challenges were not enough, there is also a steady stream of ethical and emotional questions to navigate. Some people are worried that artificial intelligence, specifically machine learning, is dangerous. What if the robots (actually, the software) become smarter than us? Won’t they take over? Are we doomed? Consider our species around 350,000 years ago. We began to use fire. Fire was (is) dangerous! However, when used properly, fire added value and helped with human evolution. The answer to “is AI good or bad?” is not a simple yes or no. Just like the use of fire, Max Tegmark, MIT Astrophysist said on the Smartless podcast, it depends on how we use AI. Artificial Intelligence is “still pretty dumb.” Getting AI to understand the information it consumes and offers back to us is still the challenge that makes this technology hard.
Consider ChatGPT: a well curated source of data (the ‘spark’ for our use of ‘fire’). But its data are not current. The tool quickly parrots answers back to those asking. It just responds with what seems like well-constructed answers. However, ChatGPT does not understand. (Please, do not get us wrong, ChatGPT is a great achievement. But it is not accurate enough to be considered reliable.)
Commenting on the reliability of ChatGPT output, Ben Thompson and James Allworth, from the Exponent podcast, describe it as a “high-schooler essay, delivered with the confidence of a 48-year-old man.” They say there is (or will be) a need for humans to learn how to verify AI-synthesized information. The challenge for organizations will be learning how to do this, how to staff for this, how to build process for this. Part of that challenge may be overcoming resistance from existing staff, who fear that AI will replace them. (We are not going to make a prediction either way on that matter; but we would observe that past technological disruptions tend to create new and diverse types of jobs even as they eliminate certain existing jobs.)
Long path
Whether applied for the greater good, or for unintended evil (see the Industrial Internet of Things article summarizing the plot of The Terminator series – and discussing AI), software that can mimic human thought is a long way off. We first need to clean and organize our data. We also need to develop rules for thought processing that replicates human thinking. We need to come together as a global community and, whether through technology or human intervention, work towards AI outputs we can trust. That time is not here.
Movies like Terminator, Ex Machina, and others spin up our imagination. Meantime, ChatGPT, Alexa, Siri, and Google Search are giving us a legitimately exciting glimpse of what is already possible with AI.
And so, the race is now on to create company-specific versions for employees and customers. Those who succeed in the AI race will be the ones who define the problems they wish to solve, set realistic expectations for what is possible as technology evolves, and acknowledge the people and process challenges they will face. This will be exciting, but not easy. Artificial intelligence is hard!